AI for
Financial Services
Bringing the Value of Self-Hosted LLMs to Financial Services
SEE Shakudo IN ACTION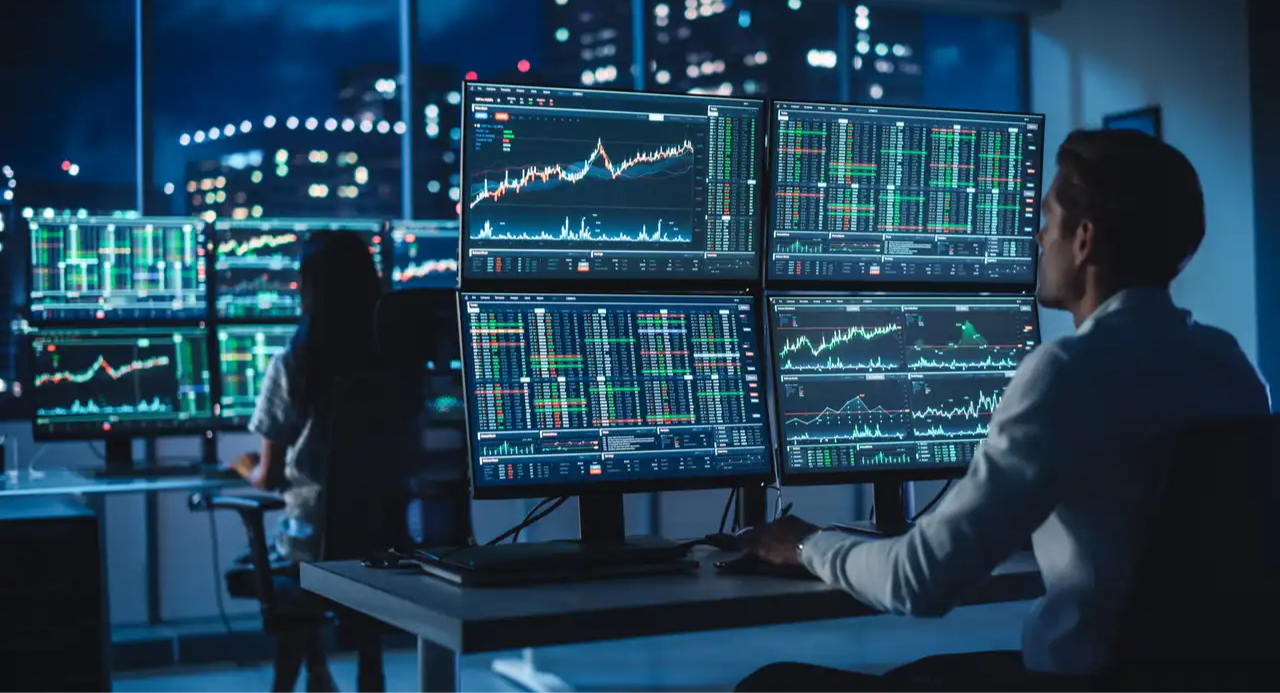
Streamline the review of earnings transcripts, expert calls, sell-side research, news, and financial filings. Extract key data points, aggregate views, and create time-series insights.
Utilize natural language interfaces to review historical notes, summarize views, and post commentary directly within your research management system (RMS).
Quickly draft responses to due diligence questionnaires (DDQs) and requests for proposals (RFPs) by leveraging prior responses and relevant fund and regulatory documents.
Prepare for LP meetings by accessing contact biographies, internal communications, and CRM activity. Interact with your CRM using natural language prompts for efficient data management.
As the landscape of alternative asset management continues to evolve, the intersection of technology and data stands as a cornerstone for innovation and maintaining a competitive edge. Many financial services are looking to external and internal data sources to capture trends, uncover information, and provide insight around operational opportunities. Large language models (LLMs) serve a growing use case around this data, as they provide an amazing ability to summarize unstructured data.
While existing models like BloombergGPT have demonstrated remarkable capabilities, they often fall short in addressing the nuances of organizational-specific use cases for LLMs. Fine-tuning improves model performance, but has its limits due to the specific training the fine-tuning is specialized for. Enter Retrieval Augmented Generation (RAG).
RAG fundamentally improves use case performance by enabling LLMs to access organizational context in real time. Whether it’s industry news, market data, recorded earnings calls, or internal emails, these data sources can provide needed context for the LLM. The Shakudo platform is a catalyst in the realization of RAG-based LLM architecture for financial services .
At Shakudo, our mission is to be more than a platform. We aim to be a strategic partner, empowering technology and data leaders in financial services to navigate the complexities of AI and data with confidence and agility.
Get in touch with the Shakudo team to learn more about how our platform can enable your fund to stand up a RAG-based LLM!