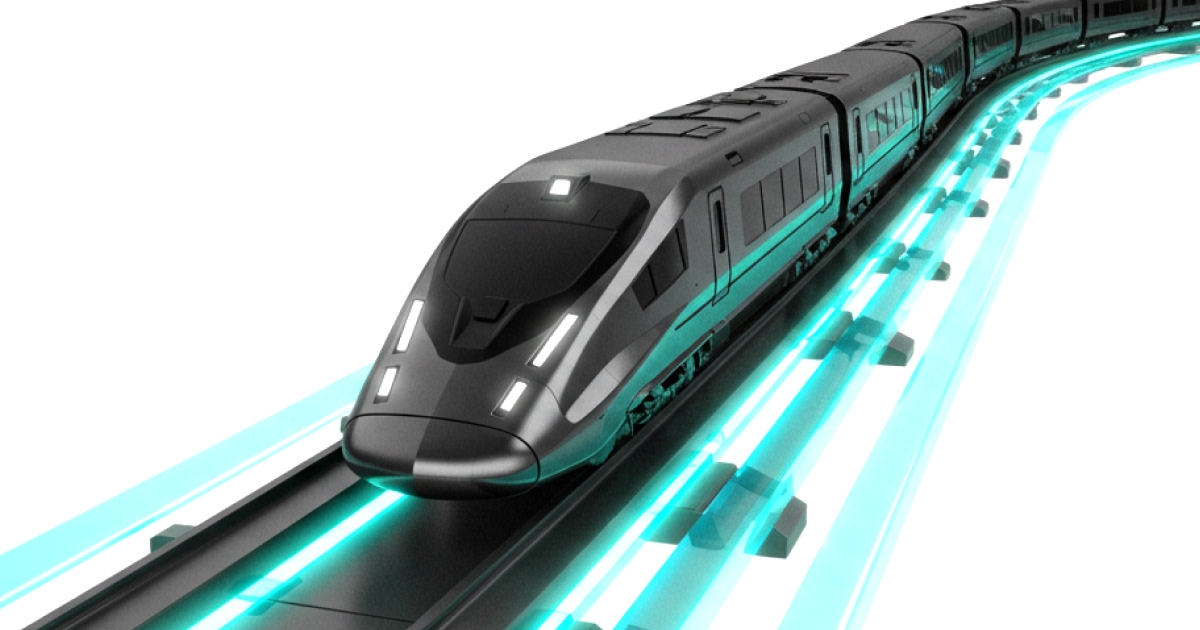
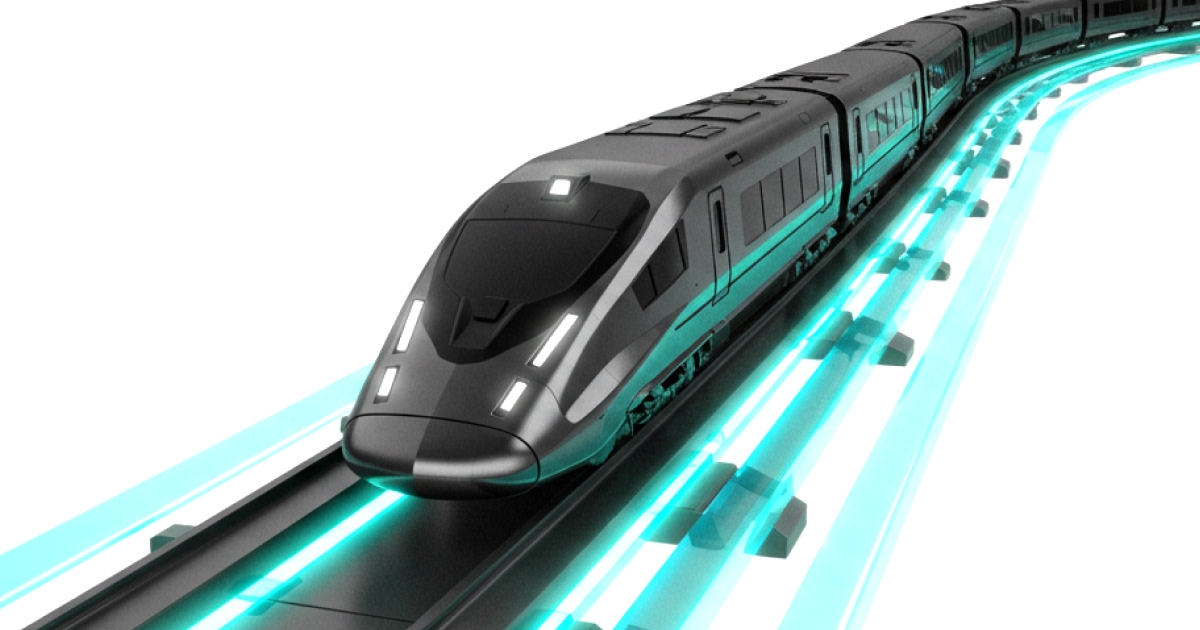
From boardrooms to engineering standups, AI innovation is on everyone’s radar right now. Still, when we speak with teams inside large organizations, there’s a common thread: while the urgency to move fast is clear, the path to doing it sustainably is anything but. True, teams are expected to operate at startup speed, yet they’re held to enterprise standards of stability, compliance, and performance. As competition intensifies, the ability to move with both speed and stability has become a critical differentiator—yet many teams remain unprepared to close that gap.
Over the past few years, Shakudo has partnered with a number of forward-thinking clients to tackle exactly this challenge: how to empower data and AI teams to move fast, stay aligned with business goals, and scale their impact.
Along the way, we’ve learned that what really sets fast-moving AI teams apart isn’t just better models or more headcount—it’s the data strategy and foundation they’re working on. The right infrastructure makes it easier to build, test, and launch ideas without getting stuck in red tape or technical debt.
Today, we’re sharing four key ways Shakudo helps fast-moving teams win with better AI infrastructure—what’s worked, what hasn’t, and what truly matters when building AI systems that can scale quickly and securely in the real world.
Clients often come to us with a bold vision: use AI to create smarter decision-making frameworks, automate repetitive processes, and provide better tools to teams across investment, operations, and strategy. But realizing that vision required overcoming familiar enterprise hurdles—legacy infrastructure, data silos, and the friction of moving AI projects from prototype to production.
Rather than building from scratch or juggling a patchwork of tools, what clients needed was an overarching operating system designed to support fast-moving data teams: something flexible enough to experiment with, yet robust enough to scale.
Shakudo provides the operating layer that empowers data teams to move from idea to impact—quickly and sustainably. The Shakudo OS combines orchestration, compute, data workflows, and collaboration features to enable technical teams to focus on solving business problems—not wrangling infrastructure.
To a lot of clients, this meant going from exploratory projects to live applications across multiple high-value areas.
Use Case: Enabling context-rich querying across a large corpus of internal knowledge and documentation.
Context: Fast-moving businesses often require quick, accurate access to institutional knowledge, and traditional keyword search falls short when navigating complex, unstructured documentation. As teams scale, the ability to retrieve meaningful context from internal data becomes critical—not just for efficiency, but for informed decision-making.
Solution: Utilizing databases like Neo4j on Shakudo, our team helped clients implement Graph-based Retrieval-Augmented Generation (Graph RAG), mapping relationships between document chunks to unlock deeper insights. This way, clients can surface relevant information from across their portfolio with precision.
This dramatically improved how internal teams accessed institutional knowledge—reducing time spent digging through documents and enhancing the relevance of insights.
Use Case: Automating extraction and interpretation of financial documents using Optical Character Recognition (OCR) and AI models.
Context: When a finance or investment team at an enterprise comes to Shakudo, their needs can be as simple as this: they want to extract insights from large volumes of unstructured financial documents—quickly and accurately. Manually reviewing these documents is time-consuming, error-prone, and difficult to scale, especially when decisions depend on timely, consistent analysis.
Impact: By comparing the output of multiple AI models, the Shakudo team replaced manual research workflows with automated pipelines that deliver faster and more consistent insights. Right away, this resulted in a more agile decision-making process, with better information available at key moments.
Use Case: Enriching market evaluations with sentiment insights derived from macroeconomic sources.
Context: Every business needs timely, data-driven insights to evaluate market conditions and adjust strategy accordingly. Traditional market evaluation methods often rely on static indicators or delayed reporting, which can miss subtle shifts in sentiment and momentum. By incorporating NLP-driven sentiment extraction from macroeconomic data sources, organizations can supplement structured models with dynamic, real-time signals—enhancing responsiveness and predictive accuracy.
Impact: Our sentiment analysis pipeline gave the client a new lens through which to assess market trends—complementing existing research tools and helping inform strategy in volatile conditions. By extracting and analyzing sentiment from macroeconomic data sources, data teams were able to complement their existing tools with automated insights—enabling faster, more confident decisions in response to shifting market trends.
Use Case: Building parallel workflows to connect proprietary internal systems to the AI layer.
Context: The fragmentation of data across internal systems has always been a major barrier to effective AI adoption. When data is locked in silos—spread across departments, tools, or legacy platforms—building cohesive, end-to-end workflows becomes difficult, if not impossible. Connecting these systems is essential for organizations looking to fully leverage their data for advanced analytics and automation.
Impact: The Shakudo team unified internal datasets and built intelligent workflows that seamlessly connect previously siloed systems. By integrating custom workflows into the Shakudo system, they unlocked advanced analytics and AI-powered automation across teams. This became a foundational step in transforming scattered data into a strategic asset—making it more accessible, actionable, and aligned with real business goals.
Our most successful engagements go far beyond technology. In this case, it was the shared commitment to iteration, transparency, and collaboration that made all the difference.
Workshops, strategy sessions, and open feedback loops allowed us to align closely with the client’s goals—and build solutions that their teams actually used. Partnerships like this show what’s possible when infrastructure supports—not slows down—innovation.
Check out how Shakudo has helped companies like Ritual and CentralReach reach their full potential.
If there’s one key takeaway from what we’ve learned in the past year, it’s this: the success of AI doesn’t just depend on algorithms—it depends on infrastructure that lets data teams build, test, and scale quickly.
That’s precisely what we deliver at Shakudo. Whether it’s connecting complex internal systems, enabling document intelligence, or supporting advanced use cases like Graph RAG and sentiment analysis, our OS is designed for fast-moving AI teams that need real results.
Are you looking to accelerate your AI initiatives and unlock the full potential of your data?
From boardrooms to engineering standups, AI innovation is on everyone’s radar right now. Still, when we speak with teams inside large organizations, there’s a common thread: while the urgency to move fast is clear, the path to doing it sustainably is anything but. True, teams are expected to operate at startup speed, yet they’re held to enterprise standards of stability, compliance, and performance. As competition intensifies, the ability to move with both speed and stability has become a critical differentiator—yet many teams remain unprepared to close that gap.
Over the past few years, Shakudo has partnered with a number of forward-thinking clients to tackle exactly this challenge: how to empower data and AI teams to move fast, stay aligned with business goals, and scale their impact.
Along the way, we’ve learned that what really sets fast-moving AI teams apart isn’t just better models or more headcount—it’s the data strategy and foundation they’re working on. The right infrastructure makes it easier to build, test, and launch ideas without getting stuck in red tape or technical debt.
Today, we’re sharing four key ways Shakudo helps fast-moving teams win with better AI infrastructure—what’s worked, what hasn’t, and what truly matters when building AI systems that can scale quickly and securely in the real world.
Clients often come to us with a bold vision: use AI to create smarter decision-making frameworks, automate repetitive processes, and provide better tools to teams across investment, operations, and strategy. But realizing that vision required overcoming familiar enterprise hurdles—legacy infrastructure, data silos, and the friction of moving AI projects from prototype to production.
Rather than building from scratch or juggling a patchwork of tools, what clients needed was an overarching operating system designed to support fast-moving data teams: something flexible enough to experiment with, yet robust enough to scale.
Shakudo provides the operating layer that empowers data teams to move from idea to impact—quickly and sustainably. The Shakudo OS combines orchestration, compute, data workflows, and collaboration features to enable technical teams to focus on solving business problems—not wrangling infrastructure.
To a lot of clients, this meant going from exploratory projects to live applications across multiple high-value areas.
Use Case: Enabling context-rich querying across a large corpus of internal knowledge and documentation.
Context: Fast-moving businesses often require quick, accurate access to institutional knowledge, and traditional keyword search falls short when navigating complex, unstructured documentation. As teams scale, the ability to retrieve meaningful context from internal data becomes critical—not just for efficiency, but for informed decision-making.
Solution: Utilizing databases like Neo4j on Shakudo, our team helped clients implement Graph-based Retrieval-Augmented Generation (Graph RAG), mapping relationships between document chunks to unlock deeper insights. This way, clients can surface relevant information from across their portfolio with precision.
This dramatically improved how internal teams accessed institutional knowledge—reducing time spent digging through documents and enhancing the relevance of insights.
Use Case: Automating extraction and interpretation of financial documents using Optical Character Recognition (OCR) and AI models.
Context: When a finance or investment team at an enterprise comes to Shakudo, their needs can be as simple as this: they want to extract insights from large volumes of unstructured financial documents—quickly and accurately. Manually reviewing these documents is time-consuming, error-prone, and difficult to scale, especially when decisions depend on timely, consistent analysis.
Impact: By comparing the output of multiple AI models, the Shakudo team replaced manual research workflows with automated pipelines that deliver faster and more consistent insights. Right away, this resulted in a more agile decision-making process, with better information available at key moments.
Use Case: Enriching market evaluations with sentiment insights derived from macroeconomic sources.
Context: Every business needs timely, data-driven insights to evaluate market conditions and adjust strategy accordingly. Traditional market evaluation methods often rely on static indicators or delayed reporting, which can miss subtle shifts in sentiment and momentum. By incorporating NLP-driven sentiment extraction from macroeconomic data sources, organizations can supplement structured models with dynamic, real-time signals—enhancing responsiveness and predictive accuracy.
Impact: Our sentiment analysis pipeline gave the client a new lens through which to assess market trends—complementing existing research tools and helping inform strategy in volatile conditions. By extracting and analyzing sentiment from macroeconomic data sources, data teams were able to complement their existing tools with automated insights—enabling faster, more confident decisions in response to shifting market trends.
Use Case: Building parallel workflows to connect proprietary internal systems to the AI layer.
Context: The fragmentation of data across internal systems has always been a major barrier to effective AI adoption. When data is locked in silos—spread across departments, tools, or legacy platforms—building cohesive, end-to-end workflows becomes difficult, if not impossible. Connecting these systems is essential for organizations looking to fully leverage their data for advanced analytics and automation.
Impact: The Shakudo team unified internal datasets and built intelligent workflows that seamlessly connect previously siloed systems. By integrating custom workflows into the Shakudo system, they unlocked advanced analytics and AI-powered automation across teams. This became a foundational step in transforming scattered data into a strategic asset—making it more accessible, actionable, and aligned with real business goals.
Our most successful engagements go far beyond technology. In this case, it was the shared commitment to iteration, transparency, and collaboration that made all the difference.
Workshops, strategy sessions, and open feedback loops allowed us to align closely with the client’s goals—and build solutions that their teams actually used. Partnerships like this show what’s possible when infrastructure supports—not slows down—innovation.
Check out how Shakudo has helped companies like Ritual and CentralReach reach their full potential.
If there’s one key takeaway from what we’ve learned in the past year, it’s this: the success of AI doesn’t just depend on algorithms—it depends on infrastructure that lets data teams build, test, and scale quickly.
That’s precisely what we deliver at Shakudo. Whether it’s connecting complex internal systems, enabling document intelligence, or supporting advanced use cases like Graph RAG and sentiment analysis, our OS is designed for fast-moving AI teams that need real results.
Are you looking to accelerate your AI initiatives and unlock the full potential of your data?
From boardrooms to engineering standups, AI innovation is on everyone’s radar right now. Still, when we speak with teams inside large organizations, there’s a common thread: while the urgency to move fast is clear, the path to doing it sustainably is anything but. True, teams are expected to operate at startup speed, yet they’re held to enterprise standards of stability, compliance, and performance. As competition intensifies, the ability to move with both speed and stability has become a critical differentiator—yet many teams remain unprepared to close that gap.
Over the past few years, Shakudo has partnered with a number of forward-thinking clients to tackle exactly this challenge: how to empower data and AI teams to move fast, stay aligned with business goals, and scale their impact.
Along the way, we’ve learned that what really sets fast-moving AI teams apart isn’t just better models or more headcount—it’s the data strategy and foundation they’re working on. The right infrastructure makes it easier to build, test, and launch ideas without getting stuck in red tape or technical debt.
Today, we’re sharing four key ways Shakudo helps fast-moving teams win with better AI infrastructure—what’s worked, what hasn’t, and what truly matters when building AI systems that can scale quickly and securely in the real world.
Clients often come to us with a bold vision: use AI to create smarter decision-making frameworks, automate repetitive processes, and provide better tools to teams across investment, operations, and strategy. But realizing that vision required overcoming familiar enterprise hurdles—legacy infrastructure, data silos, and the friction of moving AI projects from prototype to production.
Rather than building from scratch or juggling a patchwork of tools, what clients needed was an overarching operating system designed to support fast-moving data teams: something flexible enough to experiment with, yet robust enough to scale.
Shakudo provides the operating layer that empowers data teams to move from idea to impact—quickly and sustainably. The Shakudo OS combines orchestration, compute, data workflows, and collaboration features to enable technical teams to focus on solving business problems—not wrangling infrastructure.
To a lot of clients, this meant going from exploratory projects to live applications across multiple high-value areas.
Use Case: Enabling context-rich querying across a large corpus of internal knowledge and documentation.
Context: Fast-moving businesses often require quick, accurate access to institutional knowledge, and traditional keyword search falls short when navigating complex, unstructured documentation. As teams scale, the ability to retrieve meaningful context from internal data becomes critical—not just for efficiency, but for informed decision-making.
Solution: Utilizing databases like Neo4j on Shakudo, our team helped clients implement Graph-based Retrieval-Augmented Generation (Graph RAG), mapping relationships between document chunks to unlock deeper insights. This way, clients can surface relevant information from across their portfolio with precision.
This dramatically improved how internal teams accessed institutional knowledge—reducing time spent digging through documents and enhancing the relevance of insights.
Use Case: Automating extraction and interpretation of financial documents using Optical Character Recognition (OCR) and AI models.
Context: When a finance or investment team at an enterprise comes to Shakudo, their needs can be as simple as this: they want to extract insights from large volumes of unstructured financial documents—quickly and accurately. Manually reviewing these documents is time-consuming, error-prone, and difficult to scale, especially when decisions depend on timely, consistent analysis.
Impact: By comparing the output of multiple AI models, the Shakudo team replaced manual research workflows with automated pipelines that deliver faster and more consistent insights. Right away, this resulted in a more agile decision-making process, with better information available at key moments.
Use Case: Enriching market evaluations with sentiment insights derived from macroeconomic sources.
Context: Every business needs timely, data-driven insights to evaluate market conditions and adjust strategy accordingly. Traditional market evaluation methods often rely on static indicators or delayed reporting, which can miss subtle shifts in sentiment and momentum. By incorporating NLP-driven sentiment extraction from macroeconomic data sources, organizations can supplement structured models with dynamic, real-time signals—enhancing responsiveness and predictive accuracy.
Impact: Our sentiment analysis pipeline gave the client a new lens through which to assess market trends—complementing existing research tools and helping inform strategy in volatile conditions. By extracting and analyzing sentiment from macroeconomic data sources, data teams were able to complement their existing tools with automated insights—enabling faster, more confident decisions in response to shifting market trends.
Use Case: Building parallel workflows to connect proprietary internal systems to the AI layer.
Context: The fragmentation of data across internal systems has always been a major barrier to effective AI adoption. When data is locked in silos—spread across departments, tools, or legacy platforms—building cohesive, end-to-end workflows becomes difficult, if not impossible. Connecting these systems is essential for organizations looking to fully leverage their data for advanced analytics and automation.
Impact: The Shakudo team unified internal datasets and built intelligent workflows that seamlessly connect previously siloed systems. By integrating custom workflows into the Shakudo system, they unlocked advanced analytics and AI-powered automation across teams. This became a foundational step in transforming scattered data into a strategic asset—making it more accessible, actionable, and aligned with real business goals.
Our most successful engagements go far beyond technology. In this case, it was the shared commitment to iteration, transparency, and collaboration that made all the difference.
Workshops, strategy sessions, and open feedback loops allowed us to align closely with the client’s goals—and build solutions that their teams actually used. Partnerships like this show what’s possible when infrastructure supports—not slows down—innovation.
Check out how Shakudo has helped companies like Ritual and CentralReach reach their full potential.
If there’s one key takeaway from what we’ve learned in the past year, it’s this: the success of AI doesn’t just depend on algorithms—it depends on infrastructure that lets data teams build, test, and scale quickly.
That’s precisely what we deliver at Shakudo. Whether it’s connecting complex internal systems, enabling document intelligence, or supporting advanced use cases like Graph RAG and sentiment analysis, our OS is designed for fast-moving AI teams that need real results.
Are you looking to accelerate your AI initiatives and unlock the full potential of your data?