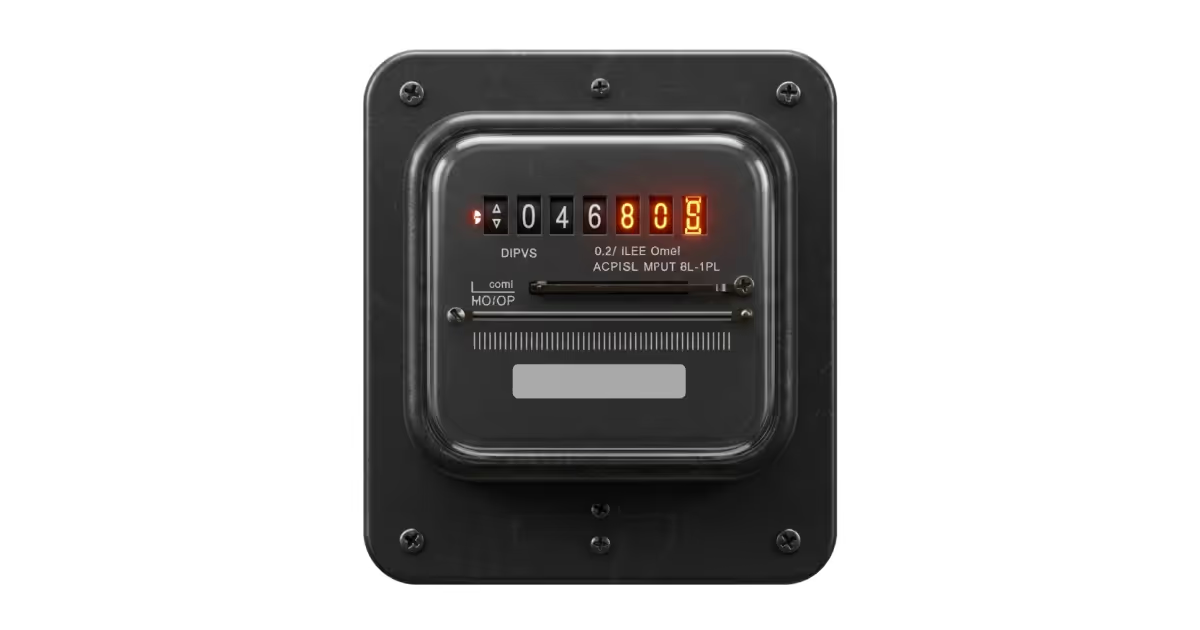
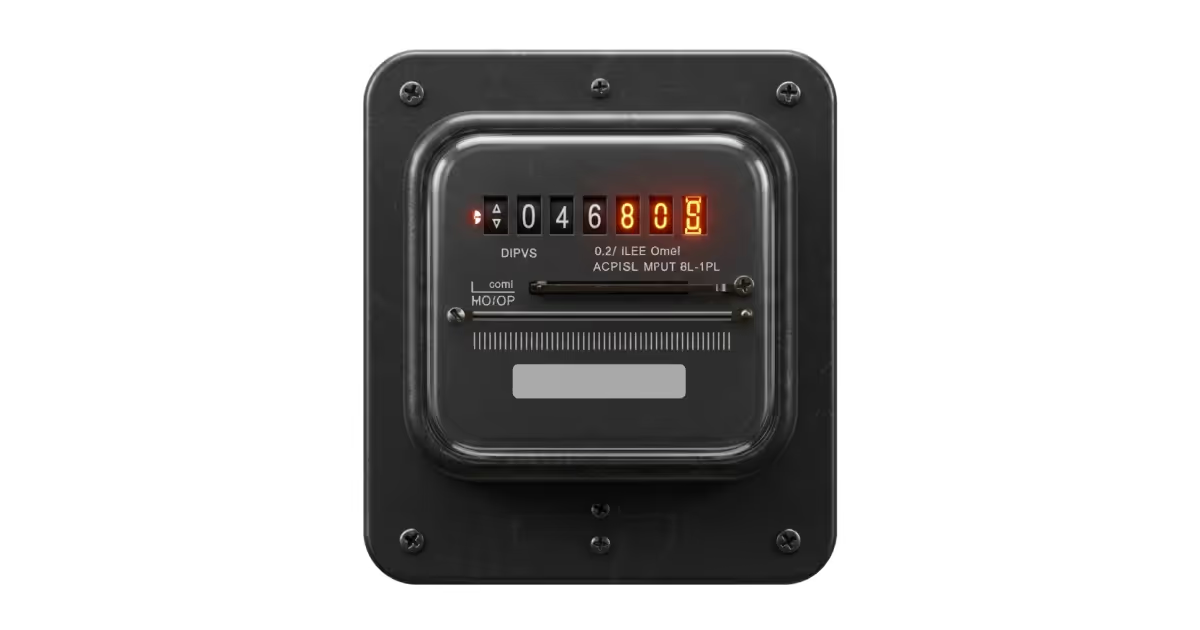
Back in 2023, in a landmark market forecast, Gartner highlighted the role of cloud computing, and predicted that by 2028, cloud computing will transition from a competitive advantage to a business necessity, with worldwide spending projected to surpass $1 trillion by 2027. Indeed, with the rapid acceleration of digital transformation, cloud computing is increasingly being used to drive innovation, disrupt markets, and scale business value—especially through the integration of AI. Its evolution from an innovation enabler to a core business function, and now to the backbone of enterprise agility and intelligence, underscores its growing role as a foundational element in modern enterprise strategy.
While many organizations have embraced cloud for its technical benefits, few have fully leveraged its role in business transformation. The cloud has evolved from a simple repository for data into an indispensable computing fabric that, when coupled with AI, is reshaping how organizations strategize, operate, and compete. Consequently, businesses are increasingly encouraged to rethink their digital strategies and modernize their core infrastructure to stay ahead of the game. From enhanced operational efficiencies and refined business intelligence applications, to secure and scalable environments that drive transformative revenue gains, this is a technology that unlocks innovation and accelerates growth.
So, how does AI shape the development of cloud computing amid the complexity of today’s digital landscape?
Today, to bring you clarity alongside the rapid pace of AI development, we dive into the top 9 ways that AI is poised to redefine cloud computing paradigms. With practical examples and key insights, we hope to give you a strategic overview for decision-making and future-proofing your organization.
AI-enhanced cloud services are redefining what’s possible in modern IT environments, particularly through dynamic resource allocation and intelligent automation. By integrating AI into cloud infrastructure, organizations can automatically adjust compute and storage resources based on real-time demand, reducing costs and minimizing downtime.
Cloud platforms now use AI to streamline deployments, manage system health, and fine-tune resource distribution across hybrid and multi-cloud environments. This level of responsiveness not only boosts operational efficiency but also enables businesses to innovate faster and scale with greater precision. Alongside optimization, AI also contributes to proactive anomaly detection, flagging potential performance issues or security threats before they escalate—enabling faster, more effective responses from IT teams.
Microsoft Azure’s AI-powered Auto-Scale feature, for example, monitors application performance and adjusts their resource allocations automatically based on predictive models. During an e-commerce sales event like Black Friday, Azure’s AI can detect increasing user activity, forecast the traffic surge, and scale out virtual machines preemptively—ensuring uninterrupted customer experiences while avoiding unnecessary overprovisioning. Ultimately, AI-enhanced cloud services empower businesses to move from reactive to proactive operations, transforming the cloud into a responsive, intelligent platform that drives agility, resilience, and continuous innovation.
In the era of generative AI, the rapid pace of model development and deployment has made Machine Learning Operations (MLOps) a critical layer in modern cloud ecosystems. AI-enabled MLOps platforms are now essential for streamlining the end-to-end machine learning lifecycle—from model training and validation to deployment, monitoring, and continuous improvement.
What sets modern MLOps apart is the integration of both proprietary and open-source models, including foundation models like GPT, LLaMA, and Claude. These platforms are increasingly incorporating advanced techniques such as prompt engineering, retrieval-augmented generation (RAG), and fine-tuning, enabling teams to build customized AI solutions with improved performance, relevance, and control.
For example, developers can use prompt engineering to tailor responses from large language models without retraining the entire model, or use RAG pipelines to ground responses in trusted data sources—improving accuracy and reducing hallucination. For seamless implementation, organizations can leverage powerful AI frameworks such as LangGraph to simplify the orchestration of complicated AI workflows and RAG pipelines.
At the infrastructure level, MLOps platforms also offer version control for datasets and models, automated pipeline orchestration, and real-time performance monitoring, enabling continuous learning and deployment in a robust, repeatable manner. These capabilities are essential in high-stakes environments like healthcare, finance, and legal services, where explainability, compliance, and auditability are non-negotiable.
The Shakudo operating system stands out as a leading example of such modern MLOps, integrating tools, data engineering, and infrastructure orchestration into one unified platform. Shakudo enables teams to plug in their preferred tools—whether open-source libraries, proprietary models, or cloud-native services—while abstracting away infrastructure complexity. Its flexible architecture supports both experimentation and production, allowing enterprises to scale AI initiatives faster while maintaining control, security, and reproducibility.
As the demand for real-time intelligence grows, Edge AI is emerging as a transformative force by bringing computation closer to where data is generated—on devices, sensors, and remote nodes. The technology can reduce latency by processing data near its source, often achieving up to a 40% reduction compared to centralized models, and can cut cloud traffic costs by as much as 70%. From autonomous vehicles to smart factories, Edge AI comes particularly handy in applications such as autonomous systems where immediacy and decentralization are critical.
On the other hand, Federated Learning complements this by enabling collaborative AI model training across distributed devices or data sources without requiring raw data to be centralized. Advanced federation techniques combine blockchain-based secure aggregation, model compression, and hybrid federated architectures to address communication overhead, data heterogeneity, and limited resources, enabling resilient and scalable cloud security systems with high efficiency.
Traditional security focuses on data at rest and in transit; however, cutting-edge innovations such as NVIDIA’s Confidential Computing now protect data during processing. Utilizing a hardware-based trusted execution environment (TEE) integrated into the H100 Tensor Core GPU, these solutions ensure that sensitive data is shielded against hypervisor and OS-level threats.
Tools like Google Cloud’s Security Command Center are drastically cutting threat response times, with self-healing infrastructures and real-time anomaly detection paving the path for proactive security management, protecting organization data while ensuring highest level of compliance with evolving regulations.
As AI workloads grow in size and complexity, enterprises are increasingly adopting multi-cloud and hybrid strategies to ensure flexibility, cost optimization, and resilience. AI is helping orchestrate and manage these complex environments, abstracting vendor-specific tools and enabling seamless transitions between providers.
Reports suggest that up to 89% of organizations now leverage distributed workloads across platforms such as AWS, Azure, and Google Cloud. Technologies like container orchestration (especially Kubernetes) and DevOps automation facilitate seamless workload migration across disparate clouds.
Contractual mechanisms, such as leaveability clauses and negotiated exit terms, further ensure agility and cost optimization as they help neutralize the high integration costs and potential delays often associated with AI cloud deployment. The push towards multi-cloud and hybrid architectures not only buffers against single-vendor dependence but also promotes innovation by fostering a competitive ecosystem where best practices and rapid deployment frameworks are continuously improved and refined.
In cloud operations, agentic AI can monitor system health, adjust compute allocations, or reroute workflows based on real-time feedback. For example, an agentic AI could identify performance degradation in a cloud service and independently migrate workloads or suggest optimizations—reducing downtime and increasing resilience.
As agentic systems mature, they will begin to collaborate with other AI systems and human teams to orchestrate high-level business goals, from optimizing infrastructure spend to ensuring regulatory compliance. This evolution marks a shift from AI as a tool to AI as an operational partner within cloud ecosystems. To explore this transformation in greater depth—including real-world applications and enterprise use cases—check out our comprehensive white paper here.
The proliferation of advanced AI models is driving the integration of generative AI into DevOps workflows. This powerful combination automates code reviews, initiates rapid vulnerability scans, and implements real-time anomaly detection, dramatically reducing exposure to zero-day exploits while enabling self-healing infrastructures.
The fusion of AI with digital asset management tools, like digital passports and model cards, streamlines threat intelligence, continuous compliance checks, and predictive analytics. Generative AI is having a major impact on DevOps, streamlining the way cloud-native applications are developed, tested, and secured. By generating code snippets, test cases, and deployment scripts, AI accelerates software delivery cycles while maintaining quality and compliance.
The integration of generative AI into DevOps workflows means teams can now develop, secure, and deploy faster—with fewer manual touchpoints and less risk. Autonomous AI coding assistants like Cline, for example, can create and modify files, execute commands, and navigate web resources while maintaining a thoughtful, permission-based approach to code generation. In the long term, autonomous pipelines where generative agents write, test, secure, and push code with minimal human input will become increasingly common.
Multimodal AI systems—those that can process and integrate text, audio, image, video, and sensor data simultaneously—are opening up new dimensions of capability in cloud applications. These models provide richer, more contextual understanding and can power next-gen experiences in everything from healthcare to smart cities.
For instance, a multimodal AI platform could analyze satellite imagery, social media text, and sensor data to assess environmental risks or monitor disaster zones in real time. Tools such as Xorbits can be implemented as a versatile library for scalable serving of language, speech recognition, and multimodal models. In cloud environments, these models can be deployed at scale and updated continuously, delivering actionable insights across domains.
By integrating multimodal AI into the cloud, enterprises can build context-aware applications that adapt to user intent, environment, and modality—enabling more human-like interactions, deeper insights, and better decision-making.
Sustainability is no longer optional—and AI is becoming a key enabler of greener cloud computing. By optimizing resource usage, minimizing energy waste, and predicting infrastructure demand, AI helps reduce the carbon footprint of data centers and cloud workloads. Here’s an overview of how AI is driving more sustainable cloud infrastructure in 2025.
For example, AI can dynamically schedule compute-intensive tasks during periods of renewable energy availability or reroute workloads to regions with lower emissions. AI-driven cooling and energy management systems also improve the efficiency of physical infrastructure, reducing operational costs and environmental impact.
As regulatory and investor pressure mounts around ESG goals, AI-powered sustainability tools will be essential in helping cloud providers and enterprises monitor emissions, report accurately, and drive continuous improvements in energy efficiency. To learn more about how to future proof your cloud with sustainable computing strategies, read our comprehensive white paper “Future-Proofing the Cloud: Sustainable Computing Strategies for Executives.”
The strategic infusion of AI into cloud computing is fundamentally reshaping modern business practices. From tangible economic benefits demonstrated through notable case studies to transformative trends in edge computing, autonomous cybersecurity, multi-cloud architectures, and sustainable operations, the landscape is evolving at an unprecedented pace.
In this rapidly changing environment, Shakudo stands at the forefront, empowering businesses to harness the full potential of AI-driven cloud solutions. With our cutting-edge platform, we deliver scalable, secure, and sustainable cloud infrastructure, helping organizations accelerate innovation, optimize performance, and stay ahead of the curve in a world where AI is the key to success.
Curious about how we can help? Click here for a personalized demo.
Back in 2023, in a landmark market forecast, Gartner highlighted the role of cloud computing, and predicted that by 2028, cloud computing will transition from a competitive advantage to a business necessity, with worldwide spending projected to surpass $1 trillion by 2027. Indeed, with the rapid acceleration of digital transformation, cloud computing is increasingly being used to drive innovation, disrupt markets, and scale business value—especially through the integration of AI. Its evolution from an innovation enabler to a core business function, and now to the backbone of enterprise agility and intelligence, underscores its growing role as a foundational element in modern enterprise strategy.
While many organizations have embraced cloud for its technical benefits, few have fully leveraged its role in business transformation. The cloud has evolved from a simple repository for data into an indispensable computing fabric that, when coupled with AI, is reshaping how organizations strategize, operate, and compete. Consequently, businesses are increasingly encouraged to rethink their digital strategies and modernize their core infrastructure to stay ahead of the game. From enhanced operational efficiencies and refined business intelligence applications, to secure and scalable environments that drive transformative revenue gains, this is a technology that unlocks innovation and accelerates growth.
So, how does AI shape the development of cloud computing amid the complexity of today’s digital landscape?
Today, to bring you clarity alongside the rapid pace of AI development, we dive into the top 9 ways that AI is poised to redefine cloud computing paradigms. With practical examples and key insights, we hope to give you a strategic overview for decision-making and future-proofing your organization.
AI-enhanced cloud services are redefining what’s possible in modern IT environments, particularly through dynamic resource allocation and intelligent automation. By integrating AI into cloud infrastructure, organizations can automatically adjust compute and storage resources based on real-time demand, reducing costs and minimizing downtime.
Cloud platforms now use AI to streamline deployments, manage system health, and fine-tune resource distribution across hybrid and multi-cloud environments. This level of responsiveness not only boosts operational efficiency but also enables businesses to innovate faster and scale with greater precision. Alongside optimization, AI also contributes to proactive anomaly detection, flagging potential performance issues or security threats before they escalate—enabling faster, more effective responses from IT teams.
Microsoft Azure’s AI-powered Auto-Scale feature, for example, monitors application performance and adjusts their resource allocations automatically based on predictive models. During an e-commerce sales event like Black Friday, Azure’s AI can detect increasing user activity, forecast the traffic surge, and scale out virtual machines preemptively—ensuring uninterrupted customer experiences while avoiding unnecessary overprovisioning. Ultimately, AI-enhanced cloud services empower businesses to move from reactive to proactive operations, transforming the cloud into a responsive, intelligent platform that drives agility, resilience, and continuous innovation.
In the era of generative AI, the rapid pace of model development and deployment has made Machine Learning Operations (MLOps) a critical layer in modern cloud ecosystems. AI-enabled MLOps platforms are now essential for streamlining the end-to-end machine learning lifecycle—from model training and validation to deployment, monitoring, and continuous improvement.
What sets modern MLOps apart is the integration of both proprietary and open-source models, including foundation models like GPT, LLaMA, and Claude. These platforms are increasingly incorporating advanced techniques such as prompt engineering, retrieval-augmented generation (RAG), and fine-tuning, enabling teams to build customized AI solutions with improved performance, relevance, and control.
For example, developers can use prompt engineering to tailor responses from large language models without retraining the entire model, or use RAG pipelines to ground responses in trusted data sources—improving accuracy and reducing hallucination. For seamless implementation, organizations can leverage powerful AI frameworks such as LangGraph to simplify the orchestration of complicated AI workflows and RAG pipelines.
At the infrastructure level, MLOps platforms also offer version control for datasets and models, automated pipeline orchestration, and real-time performance monitoring, enabling continuous learning and deployment in a robust, repeatable manner. These capabilities are essential in high-stakes environments like healthcare, finance, and legal services, where explainability, compliance, and auditability are non-negotiable.
The Shakudo operating system stands out as a leading example of such modern MLOps, integrating tools, data engineering, and infrastructure orchestration into one unified platform. Shakudo enables teams to plug in their preferred tools—whether open-source libraries, proprietary models, or cloud-native services—while abstracting away infrastructure complexity. Its flexible architecture supports both experimentation and production, allowing enterprises to scale AI initiatives faster while maintaining control, security, and reproducibility.
As the demand for real-time intelligence grows, Edge AI is emerging as a transformative force by bringing computation closer to where data is generated—on devices, sensors, and remote nodes. The technology can reduce latency by processing data near its source, often achieving up to a 40% reduction compared to centralized models, and can cut cloud traffic costs by as much as 70%. From autonomous vehicles to smart factories, Edge AI comes particularly handy in applications such as autonomous systems where immediacy and decentralization are critical.
On the other hand, Federated Learning complements this by enabling collaborative AI model training across distributed devices or data sources without requiring raw data to be centralized. Advanced federation techniques combine blockchain-based secure aggregation, model compression, and hybrid federated architectures to address communication overhead, data heterogeneity, and limited resources, enabling resilient and scalable cloud security systems with high efficiency.
Traditional security focuses on data at rest and in transit; however, cutting-edge innovations such as NVIDIA’s Confidential Computing now protect data during processing. Utilizing a hardware-based trusted execution environment (TEE) integrated into the H100 Tensor Core GPU, these solutions ensure that sensitive data is shielded against hypervisor and OS-level threats.
Tools like Google Cloud’s Security Command Center are drastically cutting threat response times, with self-healing infrastructures and real-time anomaly detection paving the path for proactive security management, protecting organization data while ensuring highest level of compliance with evolving regulations.
As AI workloads grow in size and complexity, enterprises are increasingly adopting multi-cloud and hybrid strategies to ensure flexibility, cost optimization, and resilience. AI is helping orchestrate and manage these complex environments, abstracting vendor-specific tools and enabling seamless transitions between providers.
Reports suggest that up to 89% of organizations now leverage distributed workloads across platforms such as AWS, Azure, and Google Cloud. Technologies like container orchestration (especially Kubernetes) and DevOps automation facilitate seamless workload migration across disparate clouds.
Contractual mechanisms, such as leaveability clauses and negotiated exit terms, further ensure agility and cost optimization as they help neutralize the high integration costs and potential delays often associated with AI cloud deployment. The push towards multi-cloud and hybrid architectures not only buffers against single-vendor dependence but also promotes innovation by fostering a competitive ecosystem where best practices and rapid deployment frameworks are continuously improved and refined.
In cloud operations, agentic AI can monitor system health, adjust compute allocations, or reroute workflows based on real-time feedback. For example, an agentic AI could identify performance degradation in a cloud service and independently migrate workloads or suggest optimizations—reducing downtime and increasing resilience.
As agentic systems mature, they will begin to collaborate with other AI systems and human teams to orchestrate high-level business goals, from optimizing infrastructure spend to ensuring regulatory compliance. This evolution marks a shift from AI as a tool to AI as an operational partner within cloud ecosystems. To explore this transformation in greater depth—including real-world applications and enterprise use cases—check out our comprehensive white paper here.
The proliferation of advanced AI models is driving the integration of generative AI into DevOps workflows. This powerful combination automates code reviews, initiates rapid vulnerability scans, and implements real-time anomaly detection, dramatically reducing exposure to zero-day exploits while enabling self-healing infrastructures.
The fusion of AI with digital asset management tools, like digital passports and model cards, streamlines threat intelligence, continuous compliance checks, and predictive analytics. Generative AI is having a major impact on DevOps, streamlining the way cloud-native applications are developed, tested, and secured. By generating code snippets, test cases, and deployment scripts, AI accelerates software delivery cycles while maintaining quality and compliance.
The integration of generative AI into DevOps workflows means teams can now develop, secure, and deploy faster—with fewer manual touchpoints and less risk. Autonomous AI coding assistants like Cline, for example, can create and modify files, execute commands, and navigate web resources while maintaining a thoughtful, permission-based approach to code generation. In the long term, autonomous pipelines where generative agents write, test, secure, and push code with minimal human input will become increasingly common.
Multimodal AI systems—those that can process and integrate text, audio, image, video, and sensor data simultaneously—are opening up new dimensions of capability in cloud applications. These models provide richer, more contextual understanding and can power next-gen experiences in everything from healthcare to smart cities.
For instance, a multimodal AI platform could analyze satellite imagery, social media text, and sensor data to assess environmental risks or monitor disaster zones in real time. Tools such as Xorbits can be implemented as a versatile library for scalable serving of language, speech recognition, and multimodal models. In cloud environments, these models can be deployed at scale and updated continuously, delivering actionable insights across domains.
By integrating multimodal AI into the cloud, enterprises can build context-aware applications that adapt to user intent, environment, and modality—enabling more human-like interactions, deeper insights, and better decision-making.
Sustainability is no longer optional—and AI is becoming a key enabler of greener cloud computing. By optimizing resource usage, minimizing energy waste, and predicting infrastructure demand, AI helps reduce the carbon footprint of data centers and cloud workloads. Here’s an overview of how AI is driving more sustainable cloud infrastructure in 2025.
For example, AI can dynamically schedule compute-intensive tasks during periods of renewable energy availability or reroute workloads to regions with lower emissions. AI-driven cooling and energy management systems also improve the efficiency of physical infrastructure, reducing operational costs and environmental impact.
As regulatory and investor pressure mounts around ESG goals, AI-powered sustainability tools will be essential in helping cloud providers and enterprises monitor emissions, report accurately, and drive continuous improvements in energy efficiency. To learn more about how to future proof your cloud with sustainable computing strategies, read our comprehensive white paper “Future-Proofing the Cloud: Sustainable Computing Strategies for Executives.”
The strategic infusion of AI into cloud computing is fundamentally reshaping modern business practices. From tangible economic benefits demonstrated through notable case studies to transformative trends in edge computing, autonomous cybersecurity, multi-cloud architectures, and sustainable operations, the landscape is evolving at an unprecedented pace.
In this rapidly changing environment, Shakudo stands at the forefront, empowering businesses to harness the full potential of AI-driven cloud solutions. With our cutting-edge platform, we deliver scalable, secure, and sustainable cloud infrastructure, helping organizations accelerate innovation, optimize performance, and stay ahead of the curve in a world where AI is the key to success.
Curious about how we can help? Click here for a personalized demo.
Back in 2023, in a landmark market forecast, Gartner highlighted the role of cloud computing, and predicted that by 2028, cloud computing will transition from a competitive advantage to a business necessity, with worldwide spending projected to surpass $1 trillion by 2027. Indeed, with the rapid acceleration of digital transformation, cloud computing is increasingly being used to drive innovation, disrupt markets, and scale business value—especially through the integration of AI. Its evolution from an innovation enabler to a core business function, and now to the backbone of enterprise agility and intelligence, underscores its growing role as a foundational element in modern enterprise strategy.
While many organizations have embraced cloud for its technical benefits, few have fully leveraged its role in business transformation. The cloud has evolved from a simple repository for data into an indispensable computing fabric that, when coupled with AI, is reshaping how organizations strategize, operate, and compete. Consequently, businesses are increasingly encouraged to rethink their digital strategies and modernize their core infrastructure to stay ahead of the game. From enhanced operational efficiencies and refined business intelligence applications, to secure and scalable environments that drive transformative revenue gains, this is a technology that unlocks innovation and accelerates growth.
So, how does AI shape the development of cloud computing amid the complexity of today’s digital landscape?
Today, to bring you clarity alongside the rapid pace of AI development, we dive into the top 9 ways that AI is poised to redefine cloud computing paradigms. With practical examples and key insights, we hope to give you a strategic overview for decision-making and future-proofing your organization.
AI-enhanced cloud services are redefining what’s possible in modern IT environments, particularly through dynamic resource allocation and intelligent automation. By integrating AI into cloud infrastructure, organizations can automatically adjust compute and storage resources based on real-time demand, reducing costs and minimizing downtime.
Cloud platforms now use AI to streamline deployments, manage system health, and fine-tune resource distribution across hybrid and multi-cloud environments. This level of responsiveness not only boosts operational efficiency but also enables businesses to innovate faster and scale with greater precision. Alongside optimization, AI also contributes to proactive anomaly detection, flagging potential performance issues or security threats before they escalate—enabling faster, more effective responses from IT teams.
Microsoft Azure’s AI-powered Auto-Scale feature, for example, monitors application performance and adjusts their resource allocations automatically based on predictive models. During an e-commerce sales event like Black Friday, Azure’s AI can detect increasing user activity, forecast the traffic surge, and scale out virtual machines preemptively—ensuring uninterrupted customer experiences while avoiding unnecessary overprovisioning. Ultimately, AI-enhanced cloud services empower businesses to move from reactive to proactive operations, transforming the cloud into a responsive, intelligent platform that drives agility, resilience, and continuous innovation.
In the era of generative AI, the rapid pace of model development and deployment has made Machine Learning Operations (MLOps) a critical layer in modern cloud ecosystems. AI-enabled MLOps platforms are now essential for streamlining the end-to-end machine learning lifecycle—from model training and validation to deployment, monitoring, and continuous improvement.
What sets modern MLOps apart is the integration of both proprietary and open-source models, including foundation models like GPT, LLaMA, and Claude. These platforms are increasingly incorporating advanced techniques such as prompt engineering, retrieval-augmented generation (RAG), and fine-tuning, enabling teams to build customized AI solutions with improved performance, relevance, and control.
For example, developers can use prompt engineering to tailor responses from large language models without retraining the entire model, or use RAG pipelines to ground responses in trusted data sources—improving accuracy and reducing hallucination. For seamless implementation, organizations can leverage powerful AI frameworks such as LangGraph to simplify the orchestration of complicated AI workflows and RAG pipelines.
At the infrastructure level, MLOps platforms also offer version control for datasets and models, automated pipeline orchestration, and real-time performance monitoring, enabling continuous learning and deployment in a robust, repeatable manner. These capabilities are essential in high-stakes environments like healthcare, finance, and legal services, where explainability, compliance, and auditability are non-negotiable.
The Shakudo operating system stands out as a leading example of such modern MLOps, integrating tools, data engineering, and infrastructure orchestration into one unified platform. Shakudo enables teams to plug in their preferred tools—whether open-source libraries, proprietary models, or cloud-native services—while abstracting away infrastructure complexity. Its flexible architecture supports both experimentation and production, allowing enterprises to scale AI initiatives faster while maintaining control, security, and reproducibility.
As the demand for real-time intelligence grows, Edge AI is emerging as a transformative force by bringing computation closer to where data is generated—on devices, sensors, and remote nodes. The technology can reduce latency by processing data near its source, often achieving up to a 40% reduction compared to centralized models, and can cut cloud traffic costs by as much as 70%. From autonomous vehicles to smart factories, Edge AI comes particularly handy in applications such as autonomous systems where immediacy and decentralization are critical.
On the other hand, Federated Learning complements this by enabling collaborative AI model training across distributed devices or data sources without requiring raw data to be centralized. Advanced federation techniques combine blockchain-based secure aggregation, model compression, and hybrid federated architectures to address communication overhead, data heterogeneity, and limited resources, enabling resilient and scalable cloud security systems with high efficiency.
Traditional security focuses on data at rest and in transit; however, cutting-edge innovations such as NVIDIA’s Confidential Computing now protect data during processing. Utilizing a hardware-based trusted execution environment (TEE) integrated into the H100 Tensor Core GPU, these solutions ensure that sensitive data is shielded against hypervisor and OS-level threats.
Tools like Google Cloud’s Security Command Center are drastically cutting threat response times, with self-healing infrastructures and real-time anomaly detection paving the path for proactive security management, protecting organization data while ensuring highest level of compliance with evolving regulations.
As AI workloads grow in size and complexity, enterprises are increasingly adopting multi-cloud and hybrid strategies to ensure flexibility, cost optimization, and resilience. AI is helping orchestrate and manage these complex environments, abstracting vendor-specific tools and enabling seamless transitions between providers.
Reports suggest that up to 89% of organizations now leverage distributed workloads across platforms such as AWS, Azure, and Google Cloud. Technologies like container orchestration (especially Kubernetes) and DevOps automation facilitate seamless workload migration across disparate clouds.
Contractual mechanisms, such as leaveability clauses and negotiated exit terms, further ensure agility and cost optimization as they help neutralize the high integration costs and potential delays often associated with AI cloud deployment. The push towards multi-cloud and hybrid architectures not only buffers against single-vendor dependence but also promotes innovation by fostering a competitive ecosystem where best practices and rapid deployment frameworks are continuously improved and refined.
In cloud operations, agentic AI can monitor system health, adjust compute allocations, or reroute workflows based on real-time feedback. For example, an agentic AI could identify performance degradation in a cloud service and independently migrate workloads or suggest optimizations—reducing downtime and increasing resilience.
As agentic systems mature, they will begin to collaborate with other AI systems and human teams to orchestrate high-level business goals, from optimizing infrastructure spend to ensuring regulatory compliance. This evolution marks a shift from AI as a tool to AI as an operational partner within cloud ecosystems. To explore this transformation in greater depth—including real-world applications and enterprise use cases—check out our comprehensive white paper here.
The proliferation of advanced AI models is driving the integration of generative AI into DevOps workflows. This powerful combination automates code reviews, initiates rapid vulnerability scans, and implements real-time anomaly detection, dramatically reducing exposure to zero-day exploits while enabling self-healing infrastructures.
The fusion of AI with digital asset management tools, like digital passports and model cards, streamlines threat intelligence, continuous compliance checks, and predictive analytics. Generative AI is having a major impact on DevOps, streamlining the way cloud-native applications are developed, tested, and secured. By generating code snippets, test cases, and deployment scripts, AI accelerates software delivery cycles while maintaining quality and compliance.
The integration of generative AI into DevOps workflows means teams can now develop, secure, and deploy faster—with fewer manual touchpoints and less risk. Autonomous AI coding assistants like Cline, for example, can create and modify files, execute commands, and navigate web resources while maintaining a thoughtful, permission-based approach to code generation. In the long term, autonomous pipelines where generative agents write, test, secure, and push code with minimal human input will become increasingly common.
Multimodal AI systems—those that can process and integrate text, audio, image, video, and sensor data simultaneously—are opening up new dimensions of capability in cloud applications. These models provide richer, more contextual understanding and can power next-gen experiences in everything from healthcare to smart cities.
For instance, a multimodal AI platform could analyze satellite imagery, social media text, and sensor data to assess environmental risks or monitor disaster zones in real time. Tools such as Xorbits can be implemented as a versatile library for scalable serving of language, speech recognition, and multimodal models. In cloud environments, these models can be deployed at scale and updated continuously, delivering actionable insights across domains.
By integrating multimodal AI into the cloud, enterprises can build context-aware applications that adapt to user intent, environment, and modality—enabling more human-like interactions, deeper insights, and better decision-making.
Sustainability is no longer optional—and AI is becoming a key enabler of greener cloud computing. By optimizing resource usage, minimizing energy waste, and predicting infrastructure demand, AI helps reduce the carbon footprint of data centers and cloud workloads. Here’s an overview of how AI is driving more sustainable cloud infrastructure in 2025.
For example, AI can dynamically schedule compute-intensive tasks during periods of renewable energy availability or reroute workloads to regions with lower emissions. AI-driven cooling and energy management systems also improve the efficiency of physical infrastructure, reducing operational costs and environmental impact.
As regulatory and investor pressure mounts around ESG goals, AI-powered sustainability tools will be essential in helping cloud providers and enterprises monitor emissions, report accurately, and drive continuous improvements in energy efficiency. To learn more about how to future proof your cloud with sustainable computing strategies, read our comprehensive white paper “Future-Proofing the Cloud: Sustainable Computing Strategies for Executives.”
The strategic infusion of AI into cloud computing is fundamentally reshaping modern business practices. From tangible economic benefits demonstrated through notable case studies to transformative trends in edge computing, autonomous cybersecurity, multi-cloud architectures, and sustainable operations, the landscape is evolving at an unprecedented pace.
In this rapidly changing environment, Shakudo stands at the forefront, empowering businesses to harness the full potential of AI-driven cloud solutions. With our cutting-edge platform, we deliver scalable, secure, and sustainable cloud infrastructure, helping organizations accelerate innovation, optimize performance, and stay ahead of the curve in a world where AI is the key to success.
Curious about how we can help? Click here for a personalized demo.